What is Big Data Analytics?
Big data analytics is a field that utilises advanced technologies to process, analyse and extract insights from large sets of structured or unstructured data.
As businesses and organisations generate more and more data every day, the need for professionals who can make sense of it all grows as well.
In simple terms, big data analytics involves using software tools to analyse gigantic amounts of information collected from various sources, such as social media, sensors, financial transactions, customer interactions, or any other relevant source.
The primary goal is to identify patterns and correlations that can help organisations make informed decisions about their operations, customers, or products. But how exactly does big data analytics work. We need to understand the concept of Big Data first.
What is Big Data?
We must look at what Big Data is in terms of technologies. Big Data is an umbrella term that refers to the technologies and methods we use to store, analyse, and communicate data.
The term “big data” was first coined in 2008 by Google engineer Jeff Dean, who defined it as a collection of data sets so large and complex that they cannot be processed using traditional database management tools. Since then, the concept of big data has grown rapidly. Today, we use big data analytics to make sense of terabytes of data.
To take advantage of big data, organisations need to develop new strategies for processing and using this information.
5Vs of Big Data
With the help of the 5 Vs concept (volume, velocity, variety, veracity, and value), it is possible to achieve this goal cost-effectively. Allow us to illustrate this with the help of an example from the healthcare industry.
Volume
Hospitals and clinics worldwide generate massive amounts of data; each year, 2314 exabytes of data is collected in the form of patient records and test results. All of this data is being generated at a breakneck pace, contributing to the big data velocity phenomenon.
What are the typical examples of volume in Big Data?
Customer Volume – With big data analytics, we can identify the volume of customers. We can figure out how many individual customers we can acquire or how many individual customers we should focus on.
Business Volume – With big data analytics, we can identify the volume of business transactions. We can figure out which business transactions are in a win/win/lose situation and which are in a lose/lose/lose situation.
Velocity
Healthcare data makes up a sizable portion of the data flowing through the world’s wires. That proportion will continue to grow as the Internet of Things, medical devices, genomic testing, machine learning, natural language processing, and other novel data generation and processing techniques evolve.
Certain data, such as patient vital signs in the ICU, must be updated in real-time and shown instantly at the point of care. In these instances, Laney notes, system reaction time is a critical statistic for enterprises and may serve as a differentiation for suppliers building such solutions.
Variety
Big data variety is the term given to describe the various types of data included in big data sets. This includes traditional data sources such as transaction logs and customer profiles, as well as newer sources such as social media posts and machine-generated data. The variety of big data can be a challenge for organizations, which must find ways to manage and use all this information.
Veracity
The term “veracity” refers to the accuracy and dependability of the generated data. Veracity is the quality of being true or real. In big data, veracity refers to the accuracy and completeness of the data. Veracity can be compromised when data is not accurate or incomplete, leading to erroneous conclusions and incorrect decisions. To ensure that big data is reliable and useful, organisations must ensure that the data is accurate and complete before using it in decision-making processes.
There are a few things to consider when assessing the veracity of big data. First, you need to look at the source of the data. Is it reliable? Second, you must look at the methodology used to collect and analyse the data. Was it done correctly? And finally, you need to look at the results. Are they accurate?
All of these factors are important when assessing the veracity of big data. If none are up to par, then the data may not be accurate or reliable. This could have serious implications for businesses and organisations that rely on big data for decision-making purposes.
Value
The medical industry will benefit from analysing this data because it will allow for faster disease detection, better treatment, and lower costs. This is referred to as the value of big data. However, how do we store and process all of this data efficiently? We have several frameworks available, including Cassandra, Hadoop, and Spark.
How is Big Data generated?
On any given day, one million people log onto Facebook. On YouTube, 4.5 million videos are watched each day. It is estimated that 188 million emails are sent each day. That’s a lot of information, so how do you categorise any information as “big data”?
Every month, a single smartphone user generates approximately 40 exabytes of data, which is enormous. If we multiply this number by 5 billion smartphone users, it becomes overwhelming for our brains to comprehend and process. It is difficult for traditional computing systems to cope with this volume of data.
This enormous amount of information is referred to as “big data.” Take a look at the amount of data generated on the internet every minute. Snaps are shared at a rate of 2.1 million per second. Google receives 3.8 million search queries every day.
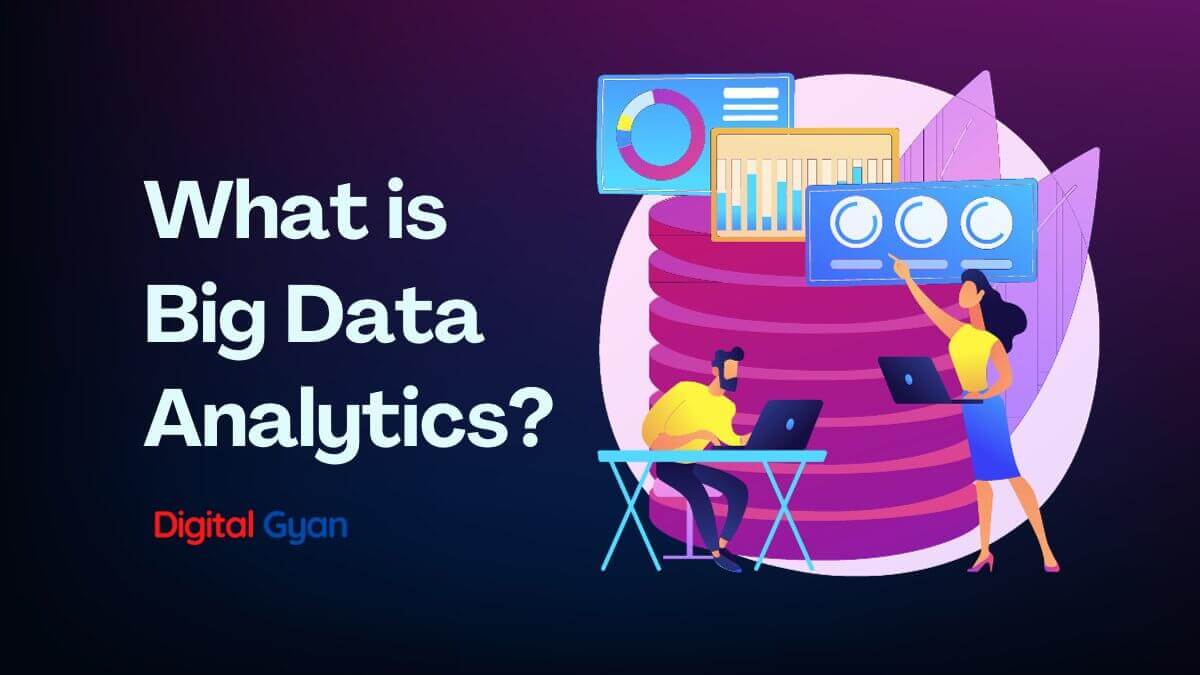
How is Big Data stored and used?
A data store may store data in typical files, databases, or even objects such as key-value stores. In any case, the fundamental principles remain unchanged: data must be accessible and usable. Accessibility provides the user with data access via programmes and tools. The data’s usability enables its effective consumption by various applications.
Storage
Data Warehouses: Traditional databases struggle with big data’s size and complexity. Data warehouses store structured data (organized in rows and columns) in a format optimized for querying and analysis.
Data Lakes: Unlike data warehouses, data lakes store all types of data, including unstructured data (text, images, audio, video) in its native format. This flexibility allows for future analytics possibilities as data science techniques evolve.
Cloud Storage: Cloud platforms offer scalable storage solutions to handle the ever-growing amounts of data.
Data Processing and Analysis
Hadoop: An open-source framework using distributed processing across clusters of computers, making it efficient for handling massive data sets.
Data Technologies: Specialized tools and data technologies are used to process and analyze big data. This includes data wrangling, transformation, and integration to prepare the data for analysis.
Using Big Data
Data Scientists who are experts and skilled in managing and analyzing big data to extract insights use big data for various purposes, including:
Predictive modeling: Identifying patterns and trends to predict future events or customer behavior.
Real-time analytics: Analyzing data streams from sources like social media or sensors to gain real-time insights.
Personalized experiences: Using data to personalize marketing campaigns, product recommendations, and customer service interactions.
What is Big data Analytics?
Big data analytics is the process of examining and analyzing large data sets that include data of various types, also known as big data. This big data comes from a multitude of data sources including social media posts, financial transactions, sensor data (from machines and IoT devices), video recordings, and even biological data. Traditional data management and analysis tools struggle with the complexity, volume, and velocity (how fast the data comes) of big data.
Big data analytics requires significant resource management for processing big data. Advanced analytics techniques are then applied to analyze the data and identify patterns that would be invisible to traditional data. These insights big data provides can be used for predictive analytics, personalised experiences, and real-time decision-making.
Is big data a good career option?
I think big data is a good career because of the huge demand there is for it. Big data is a fascinating field, and we know there is an enormous shortage of data scientists. What does that mean for you? That the demand for big data specialists is only set to grow.
Big data analytics jobs are generally in high demand because so many different types of jobs can benefit from the technology. It means a ton of money is being made through the different big data analytics projects.
For example, if you wanted to work on a project that helps determine the average time people spend shopping on Amazon, you would have to hire someone to work on that project. These people can then use the data they have to help companies learn more about what people are buying online. Companies can then decide whether they want to offer a promotion for a product that is on sale, or they can promote an extra coupon.
Summary
Now you know that Big Data is a type of data. Big data is any type that is too big, too complicated, too diverse, and too “fast-moving” to store, manipulate, and analyse using current databases or software tools.
Even within the field of data science, analytics is utilised in every industry segment. Big data can help you identify your brand’s most effective marketing channels, allowing you to judge what’s working. Data can help you provide better customer service and enhance the customer experience. Data can also help companies in decision-making by giving you insights into customer behaviour. Big data analytics jobs are generally in high demand because so many different types of jobs can benefit from the technology.
With the current development of information technology, data collection has become prevalent. Big data is too large to be processed by conventional information technology (IT). Big data has become the core of various applications and is recognised as the future of information technology. The concept of big data has expanded to include data storage and retrieval technology.